What is Epidemiology?
Epidemiology: Study of the distribution and determinants of health-related states or events in a specific population and the application of results to control health problems. Epidemiologist want to know what causes disease; how does disease spread; what can prevent disease and/or keep a population mentally, socially, and physically healthy; and what can be done to control disease.
- Distribution: Frequency and pattern of health outcomes or exposures in a population.
- Frequency: Number of occurrences.
- Example: The number of HIV cases in Fresno County.
- Pattern: Occurrence of the health outcome by time (when), place (where), and person (who).
- Trend: An upward or downward change in frequency.
- Disease trends may also remain static or unchanged.
- Determinant: Causes or factors that influence the occurrence of disease or health outcomes.
- Risk Factor: Something associated with an increase in the occurrence of a disease or outcome.
Population at Risk: A group of people that could be counted as a case if they had the disease being studied.
Public Health Surveillance: Ongoing systematic collection, analysis, interpretation, and dissemination of health data. Used to gain a better understanding of the pattern of a disease in a community in order to help control or prevent the disease.
What are some frequently used statistical terms?
Chi-square Test: Test of statistical significance.
- Chi-square goodness-of-fit test looks at one variable and compares what we would expect versus what we observed. So it looks at the difference between what is observed in the data and what we would expect if the exposure was not associated with the disease. If the difference is large, it provides evidence that there is something occurring.
- Example: Is the distribution of car accidents related to time of day? So in this case the researcher is testing to see if the observed number of car accidents per day is the same as the expected number.
- Chi-square test for independence looks to see if two categorical variables are related in a population.
- Example: A researcher wants to look into if having a parent that smokes (variable 1) is associated with teen smoking behaviors (variable 2). So in this case the researcher is testing to see if teen smoking status is or is not independent from parent smoking status.
Confidence Interval (CI) Estimate: Estimated range that is likely to contain the true population parameter of interest with a specified degree of probability (assuming no bias or confounding). CI is based on the point estimate, desired level of confidence, and sampling variability and shows the likely range of the true unknown parameter of interest. The wider the confidence interval, the less precise the estimate, and possibility of a greater amount of random error in the estimate.
- 95% Confidence Level: If the same population was sampled multiple times, the resulting confidence intervals would include the true population parameter on average approximately 95% of the time (assuming there was no bias or confounding).
- Population Parameter: Number/value that describes the population. There are several things that can be a population parameter of interest related to health outcomes. Examples include but are not limited to: mean, mean difference, proportion, rate, risk differences, and odds ratio.
Confidence Limit: The minimum and maximum value of a confidence interval.
Frequency Measures: Compare one section of a distribution to another part of the distribution or to the whole distribution.
- Rate: Frequency that an event/outcome/disease occurs in a population over a specific period of time. Rates take into consideration population size so are useful when comparing disease frequency between different population groups, time periods, or locations.
- Note: A rate has a time dimension; whereas, a proportion does not.
- Ratio: A value divided by another value.
- Example: Ratio of people in a city to number of health clinics.
- 400 clinics/ 3,000,000 people = 0.00013 clinics per person x 10,000 = 1.3 clinics per 10,000 persons or 0.00013:1
- Proportion: Type of ratio where the numerator (top number) is included in the denominator (bottom number).
- Example: Proportion of women in a study with breast cancer. Women with breast cancer in the study / Total number of women in the study (women with breast cancer + women without breast cancer)
Frequency Distributions:
- Central location: Where the distribution peaks.
Common measures of central location:
- Mean: Average of all observations. To calculate, add up the values of all observations and divide by the number of observations.
- Median: Middle value. Sort all observations in ascending order and find the value in the middle of all the observations.
- Mode: Value that occurs most frequently. To calculate arrange values into frequency distributions and identify value that occurs most frequently.
- Spread: How wide is the dispersion on both sides of the peak.
Common measures of spread:
- Range: Difference between the largest and smallest number. Range tells you the spread of values but does not tell you about the distribution of the values.
- Standard Deviation (SD): Measure of the dispersion of a frequency distribution. Basically it tells you how spread out the values are from the mean. A SD of 0 means all values are the same. If the SD is small, values are tightly grouped together and the curve of a graph is steep. If the SD is large, values are spread wide apart and the shape of the curve will be more flat.
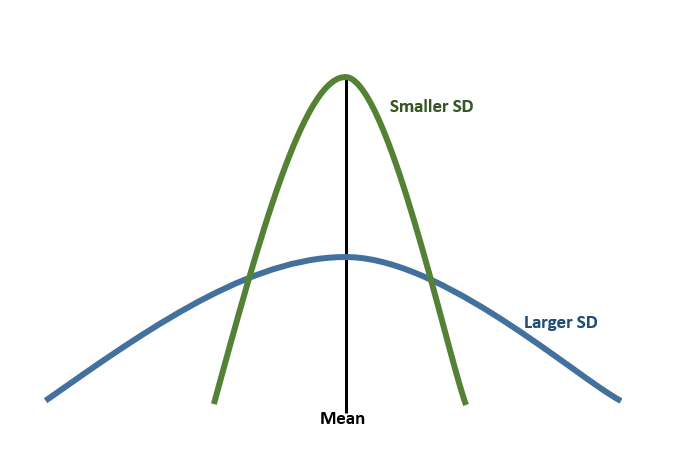
- 1 SD away from the mean (in either direction) accounts for around 68% of the group. If a curve is flatter then the SD will have to be larger to fit all 68%.
- 2 SD away from the mean (in either direction) accounts for about 95% of group.
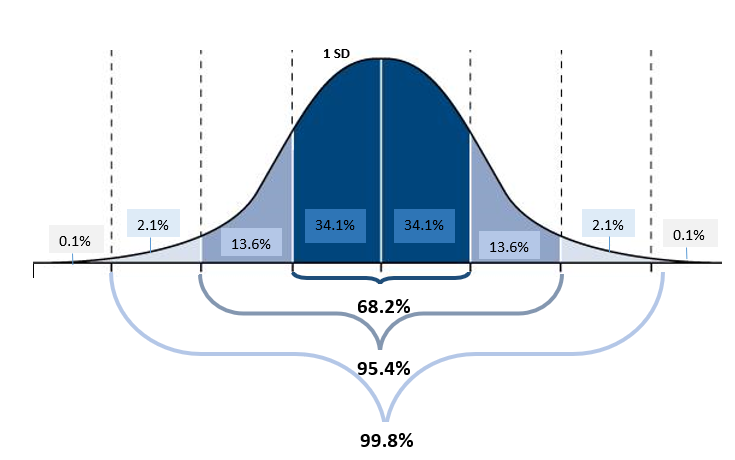
Multivariable: Two or more variables involved.
Percentile: Set of numbers from 0 to 100 that divide a distribution into 100 parts. For example, 5th percentile= point where 5% of observations are below it and 95% are above it.
Standardized Rates/ Standardization: A method that helps make it so you can compare a summary measure across different groups. For example, City A has a large retired population and City B has many college students. If comparing crude death rates for the two cities, City A’s rate may be higher simply because it has an older population. Standardization can be used to find a rate for the two cities that is comparable by adjusting for age and reducing age’s effect on the rate.
Statistically Significant: Used to determine if a study finding is the result of a true association between factors being studied or just a result of chance. If statistically significant, it suggests that the differences in the results did not occur by random chance.
- p-value: Statistical measure to show the probability that an observed difference is attributed to chance alone. Tells the researcher the probability that the test would have been at least as large as the observed value if all model assumptions were correct. So a p-value tells us how likely it is that the observed result would occur when there is no real association between the exposure and disease.
- A smaller p-value means the less likely the observed difference occurred by chance alone. Typically, a p-value of less than 0.05 is considered a statistically significant difference.
Variable: Any characteristic being measured.
- Dependent Variable: Typically, the disease or outcome variable. The variable in a study that is dependent on or effected by the exposure or independent variable.
- Independent Variable: Typically, the exposure variable or risk factors being observed or measured to see if it influences the dependent variable.
How can you measure a disease and health outcomes in a community/population?
Morbidity: Any departure from a state of physiological or psychological well-being.
Measures of Morbidity / Measures of Disease Frequency
- Attack Rate: Typically used with outbreaks and is similar to incidence rate.
Number of people at risk who develop the illness divide by the total number of people at risk. For example, the attack rate for a bad batch of clams would be the number of people who ate the clams and became ill divide by the total number of people who ate the clams.
- Incidence: Number of new cases (incident cases) of a disease occurring during a specific period of time in a population at risk for developing the disease. Tells you how many people are developing the disease. Incidence is the number of NEW cases in a time period divided by the number of people at risk of developing the disease in the same time period.
- Cumulative Incidence Proportion: Risk of acquiring a disease. Tells you the probability of developing the disease over a specified period of time. Incidence proportion estimates risk and must specify a time period. Technically incidence proportion is not a rate, it is the proportion of an “at risk” group that will develop a disease during a time period. The number of new cases over a specified period of time divided by the total number of people in the population at risk of becoming a new case at the beginning of the time period.
- Example: # of new syphilis cases reported in Fresno County in 2018 x multiplier
Fresno County population at risk in 2018
*Typically the denominator is just an estimate based on last census
- Incidence Rate – A rate must include a dimension of time. It measures the number of new cases per unit of time (“person-time”). The incidence rate is the number of new cases during a specific period of time divided by the total disease free person-time seen in the population at risk.
- Person-Time: “Person-Time” is an estimate of the actual time at risk. Most frequently the “person-time” used is “person-years” but can also be days or months. For example, in a study on the rate of stroke, a research follows 5 patients from baseline (start of study) for 5 years. The graph below shows how many years each subject remained in the study as a non-case (no stroke). “Person-Time” is the sum of the total disease-free time contributed by all subjects. The total “person-years” for this study was: 1+5+2+5+4 =17 “person-years”. 17 “person-years” would be used as the denominator for the incidence rate.
|
Year 1
|
Year 2
|
Year 3
|
Year 4
|
Year 5
|
Time at risk/ Disease-free years
|
Patient 1
|
XXXXXXXXX
|
Stroke
|
|
|
|
1 year
|
Patient 2
|
XXXXXXXXX
|
XXXXXXXXX
|
XXXXXXXXX
|
XXXXXXXX
|
XXXXXXXX
|
5 years
|
Patient 3
|
XXXXXXXXX
|
XXXXXXXXX
|
Stroke
|
|
|
2 years
|
Patient 4
|
XXXXXXXXX
|
XXXXXXXXX
|
XXXXXXXXX
|
XXXXXXXX
|
XXXXXXXX
|
5 years
|
Patient 5
|
XXXXXXXXX
|
XXXXXXXXX
|
XXXXXXXXX
|
XXXXXXXX
|
Stroke
|
4 years
|
X= Disease Free
|
- Prevalence: Frequency of existing disease. In general tells you how many people in the population have the disease, or more specifically the probability that a member of the population has the disease at a point in time. Prevalence helps us to understand the disease burden on a population. The numerator for prevalence is the number of disease cases (e.g. new and old cases) in a population at a point in time or during a period of time.
- Cumulative prevalence: Risk of ever having the disease.
- Example question: Have you ever had the flu?
- Period prevalence: Total number of people with a disease/outcome during a particular period of time divided by the total population at the midpoint of the period of time.
- Example question: Have you had the flu sometime during the last year?
- Point prevalence: Total number of people with a disease/outcome divided by the total number of people in the population at a specific point in time at risk of having the disease/outcome.
- Example question: Do you currently have the Flu?
Mortality: Death.
Measures of Mortality
- Case-Fatality Rate (CFR): Proportion of persons with a condition/disease (case) who die from the condition/disease. This tells you information about the severity of the disease by describing the percentage of fatal cases. The CFR is the number of cases who died from the condition during a period of time divided by number of incident cases during that time (i.e. number of disease cases diagnosed during that time period) multiplied by 100 to provide a percentage.
- Example: There were 300 cases of disease X in Fresno County in 2019 and 10 died.
- 10 deaths x100 = 3.3%
- 300 cases
- Mortality Rate: Measure of the number of deaths in a population for a specific period of time (typically deaths per 100,000 persons per year). Can be all cause mortality (total deaths) or cause specific mortality (deaths due to a specific cause such as Heart Disease).
- Examples: Fresno County 2019 mortality rate from all causes (per 100,000 persons)=
- Total # of deaths in Fresno County in 2019 x 100,000
- Total # of persons in the population (midyear)
- Fresno County 2019 mortality rate from Heart Disease (per 100,000 persons)=
- Total number of deaths from Heart Disease in Fresno County in 2019 x 100,000
- Total number of persons in the population (midyear)
- Infant Mortality Rate: Ratio of the number of deaths among children under 1 year of age during a period of time divided by the number of births reported during that period of time. Typically shown as the rate per 1,000 live births.
- Proportional Mortality: Proportion of deaths due to a specific cause (percent).
- Example: Proportional mortality from Heart Disease in 2019 for Fresno County=
- # of deaths from Heart Disease in Fresno County in 2019 x 100
- Total # of deaths in Fresno County in 2019
- Years of Potential Life Lost: Measure of the impact of premature death or “early death” on a population.
Measures of Births
- Birth rate: Births in a specific year divided by the mid-year population.
Other epidemiological measures of risk (relative risk, odds ratio, etc.) described in next question.
How to compare risk among two or more exposure groups/ What are measures of association?
Association: Statistical relationship between two or more variables. Important to note “association does not imply causation”, meaning just because there is a relationship or correlation between two variables, does not mean one variable causes the other.
Risk: Probability or likelihood that an event will occur.
Estimating Risk/ Measures of Probability:
- Attributable Risk: Helps answer how much of the outcome/disease that occurs in a population can be attributed to a certain exposure. Difference between the incidence of an outcome in an exposed group and the incidence of the outcome in an unexposed group.
- Odds Ratio (OR): OR shows the odds that an outcome will occur given an exposure compared to the odds the outcome will occur without the exposure. It is related to relative risk but is not the same. ORs are used when incidence rates are not know making it so relative risk cannot be calculated, such as in a case control study or cross-sectional study.
- OR of 1: no effect by the exposure (i.e. odds is the same in exposed and unexposed).
- OR greater than 1: odds of exposure among cases is greater than odds of exposure among controls (i.e. the exposure is a risk factor).
- OR less than 1: odds of exposure among cases is lower than odds of exposure among controls (i.e. the exposure is a protective factor).
- Relative Risk/Risk Ratio (RR): Comparison of the risk of a health related event/disease in two groups. Ratio of risk of disease among exposed to risk among unexposed. Disease incidence among exposed divided by disease incidence among those not exposed.
- RR of 1: no effect by the exposure (i.e. risk is the same in exposed and unexposed).
- RR greater than 1: risk of disease in exposed is greater than risk in unexposed (i.e. the exposure is a risk factor).RR less than 1: risk of disease in exposed is less than the risk in unexposed (i.e. the exposure is protective).
- Odds Ratio vs Relative Risk: The difference between odds ratio and relative risk is basically OR is the ratio of odds and RR is the ratio of probabilities.
|
Disease
|
Total (a+b)
|
Risk (a/[a+b])
|
RR
|
Odds (a/b)
|
OR
|
|
Yes (a)
|
No (b)
|
Exposed
|
40
|
60
|
100
|
40/100=0.4
|
.4/.10= 4
|
40/60=0.7
|
.7/.11=6.4
|
Unexposed
|
10
|
90
|
100
|
10/100=0.10
|
10/90=0.11
|
How can you compare disease rates?
Rate: A measure of frequency in a defined period of time (a time dimension is required for it to be a rate). A rate tells you how fast the disease is occurring.
Age-Adjusted Rate: A rate modified to eliminate the effect of different age distributions in different populations. This adjustment makes it so you can compare two or more populations with different age distributions.
Age-Specific Rate: A rate that is limited to a particular age group.
Crude Rate: Total rate for an entire population in a period of time. This rate is not limited to a particular group or adjusted for any subgroups in the population.
Proportion: Type of ratio in which the numerator is included in the denominator. A proportion tells you what fraction of the group the disease affects.
What are measures of error and bias?
Bias: Any trend in the collection, analysis, interpretation, publication, or review of data that can lead to a conclusion that is systematically different from the truth.
Confounding: When the effect of the exposure on an outcome is mixed with the effect of another factor (confounder). Making it so the association between the risk factor and disease can be explained by a third factor associated with both the disease and the risk factor. For example, an alcohol and lung cancer association may be confounded by smoking since those who smoke are more likely to drink alcohol and smoking is associated with lung cancer.
- Adjusted Odds Ratio (OR)/ Adjusted Relative Risk (RR): Adjusts for confounders/takes into consideration other variables to provide a more valid estimate of risk.
- Crude OR/RR: No adjustment/unadjusted OR/RR.
Validity: The degree a measurement actually measures or detects what it is supposed to measure. Different from reliability. For example, the validity of a skin cancer screening test tells us the tests ability to distinguish between who has skin cancer and who does not have skin cancer.
Reliability: Consistency of a measurement. If you repeat a measurement/test in the same subjects will you get the same result. Different from Validity.
· Difference between Validity and Reliability: Reliability tells us if repeated would the result be the same; whereas, validity tells us if the measurement is valid/true.
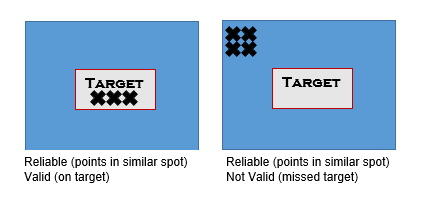
What are the different types of studies?
Role of Researcher:
- Experimental Study: Researchers control the exposure. For example, when the researcher randomly selects half of subjects to receive a new treatment and the other half (controls) to receive the current standard of care.
- Quasi-experimental: These types of studies do not use randomization to assign exposure status (treatment or control); subjects are selected for exposure from pre-existing groups. These studies have less internal validity than a randomized experiment.
- Observational Study: When researchers do not control the exposure. For example, researchers look at the risk of lung cancer in smokers. Researchers do not assign people to smoke but instead observe what happens to people who decided to smoke.
Purpose of Study:
- Descriptive Epidemiology: Answers questions about health related to who, what, when, and where.
- Analytic Epidemiology: Answers questions about health related to how and why.
Analytic Study Design Types:
- Case-Control Study: Study of those with the disease and those without. Observational study that starts with the researcher/investigator identifying groups (cases and controls) by an outcome/disease of interest: do participants have (case) or do not have (control) a disease/outcome. Then looking backward to establish the level of exposure. For case-control studies, researchers determine the proportion of cases with the exposure, cases without the exposure, controls with the exposure, and controls without the exposure.
- Control: In a case-control study, a control is a person without the disease or outcome of interest.
- Cohort Study: Study that follows subjects over time. Observational study that starts by the researcher/investigator selecting groups by their exposure status (exposed individuals and non-exposed individuals) and then following the groups over time to see if an outcome develops (e.g. disease). There can be more than 2 groups.
- Cross-Sectional Study: Study that provides a “snap-shot” of a population. Observational study that measures the outcome/disease and exposure at the same time.
- Ecological Study: Study where the data is analyzed at the group level instead of individual level. Observational study that involves a comparison of disease frequency between populations in relation to one or more risk factors. Comparisons are made at a group level because risk factors are not known for individuals.
- Example: Comparing the incidence of skin cancer (disease/outcome) in the United States to the incidence in Australia by UV level (risk factor/exposure).
- Ecological Fallacy: Ecological studies do not know the risk factor information at an individual level but only a population level. Meaning results cannot be applied to an individual. The ecological fallacy occurs when inferences are incorrectly made about an individual based on an ecological study/aggregated data for a group.
- Inferences: Conclusions based on reasoning.
- Aggregated data: Combined or grouped data.
- Randomized Control Trial (RTC): Experimental study where the researcher controls the exposure to subjects. Whether a subject gets the exposure or not is based on randomization. Subjects are randomly assigned to the experimental group or control group.
- Randomization: Process of randomly assigning subjects to an exposure group (exposed or unexposed).
- Control: In a RCT, the control group is the group who does not receive the experimental exposure.
- Systematic Review: Researcher focuses on a research question, reviews previous studies on the topic, evaluates the quality of each study, and summarizes the high quality studies.
- Meta-Analysis: A type of systematic review that combines the data/results of multiple different studies on the same topic and combines them into one large sample. Studies are weighted during a secondary statistical analysis of outcomes.
What are some terms to describe a disease event?
Cluster: A group of cases in a specific time or place that may or may not be greater than the expected rate.
Endemic: Occurrence of a disease in a population at ‘normally’ expected levels. An endemic disease occurs at a constant level in an area or population.
- Example: Malaria is endemic in India.
Epidemic: Occurrence of a disease in a population at levels above what is ‘normally’ expected in a population over a period of time.
- Outbreak Investigation: Investigation of the cause and control of an epidemic or outbreak in a specific location or population of people.
Pandemic: Epidemic that is over a large area, typically an epidemic that crosses international borders.
- Examples of past pandemics: The “Black Death” plague stretched from Asia to Europe and killed more than 75 million people. The “Spanish” flu of 1918 killed 40-50 million people from all over the world.
What are frequent terms associated with disease transmission?
Communicable Disease: Infectious disease that can be transmitted from an infected person, animal, or reservoir to a susceptible host.
- Disease Reservoir: A living (e.g. person, animal, or plant) or nonliving site (e.g. soil or water) where an infectious agent/disease naturally lives and multiples.
- Example: A bat with rabies or Legionnaires in a water-cooling tower.
Contact: A person who has been exposed to an infectious disease.
Direct Transmission: An infectious disease spreading through direct contact. Direct person-to-person contact: an ill person touches or exchanges body fluids with another person (this can be done by an ill person coughing or sneezing near/on someone).
- Example of direct transmission: A person gets the flu from their significant other during a kiss.
- Example of indirect transmission: An ill person coughs into their hand and then touches a doorknob. Later a second person touches the same doorknob, then touches their face, and becomes infected.
Disease Vector: A carrier of disease that transmits the disease to another living organism.
- Examples: Mosquitos are a vector for West Nile Virus and ticks are a vector for Lyme disease.
Exposure: Having a feature that is being studied. Exposures can be medication use, diet, genetics, employment, behaviors, occupation, etc.
- Examples of possible “exposures”: smoking, eating a high fat diet, working outside, playing football, etc.
Herd Immunity: Group protection from the spread of an infection based on a high proportion of individuals within a group with resistance. So if a large percentage of the population is immune/resistant to the disease, the entire population is protected. For example, the more people with measles vaccinations in a group, the less ability measles has to spread among the group. Those who are vaccinated stop the disease from spreading, protecting those who have not yet been or cannot be vaccinated.
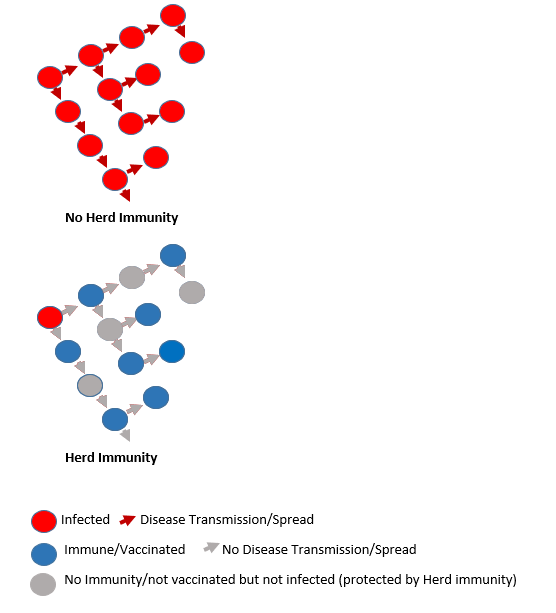
Host: A person or other animal that harbors an infectious agent.
Incident case: Newly acquired case of a disease or outcome.
Incubation Period: Period of time between exposure and when disease signs and symptoms appear.
Infectious Period: Period of time when the infected can transmit or spread the infection to others.
These questions and responses were created by Dr. Koch-Kumar, Senior Epidemiologist (May 2019). If you have additional questions please refer to the Contact Information on the Epidemiology Program main page.